Plenary Speakers
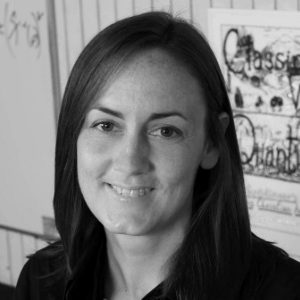
Quantitative comparisons between models and data to provide new insights in cell and developmental biology
Abstract:
Simple mathematical models have had remarkable successes in biology, framing how we understand a host of mechanisms and processes. However, with the advent of a host of new experimental technologies, the last ten years has seen an explosion in the amount and types of quantitative data now being generated. This sets a new challenge for the field – to develop, calibrate and analyse new models to interpret these data. In this talk I will use examples relating to cell motility and proliferation to showcase how quantitative comparisons between models and data can help tease apart subtle details of biological mechanisms.
Bio:
Ruth Baker is Professor of Applied Mathematics at the Mathematical Institute, University of Oxford. Her research sits at the interface of applied mathematics and the biomedical sciences. In particular, it focusses on the development and application of technologies to transform understanding of how cell-level biomechanical and biochemical mechanisms drive morphogenesis at the cell and tissue level. The tools she develops to analyse models draw upon ideas from a diverse range of subfields of mathematics and, importantly, theoretical developments are always motivated by, and embedded within the study of particular biological systems.
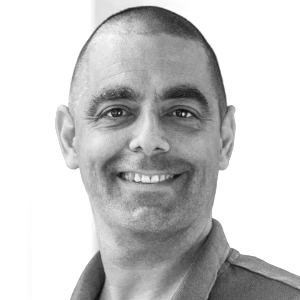
Executable Digital Twins - Integrating the digital and real world
Abstract:
We live in a world of exploding complexity with enormous challenges. Digital twins, tightly integrating the real and the digital world, are a key enabler for decision making in the context of complex systems. While the digital twin has become an intrinsic part of the product creation process, its true power lies in the connectivity of the digital representation with its physical counterpart. To be able to use a digital twin scalable in this context, the concept of an executable digital twin has been proposed. An executable digital twin is a stand-alone and self-contained executable model for a specific set of behaviors in a specific context. It can be leveraged by anyone at any point in lifecycle. To achieve this, a broad toolset of mathematical technologies is required - ranging from model order reduction, calibration to hybrid physics- and data-based models. In this presentation, we review the concept of executable digital twins, address mathematical key building blocks such as model order reduction, real-time models, state estimation, and co-simulation and detail its power along a few selected use cases.
Bio:
Dirk Hartmann is an industrial mathematician, Siemens Technical Fellow, intrapreneur, and thought leader in the field of Simulation and Digital Twin. Multiple of his innovations have led to novel products and services and have been showcased at top-level innovation events. Among many distinctions, he received the prestigious Werner-von-Siemens Top Innovator award 2019 for his life-time achievements and the Siemens Inventor of the Year 2021 award.
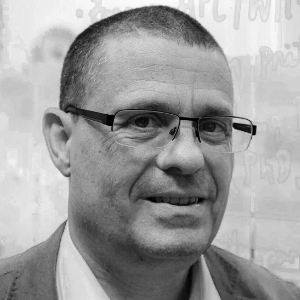
Taylor Mapping and Polynomial Neural Networks for solving forward and inverse Ordinary Differential Equations
Abstract:
Companies have a continuous need to drive their business forward by developing new products or by automating production process. Digital Twins refer to virtual simulation models of components or products which can imitate the use and properties in real time. The models also authentically simulate the product’s natural user environment and its handling. Therefore, the development of technical components by digital methods and equipping the components with additional functions is becoming increasingly important. Many of the models are based on ordinary differential equations (ODEs) and most of the technical problems are inverse problems that are difficult to solve. One the one hand, they often contain parameters that are only partly unknown, e.g., material parameters. On the other hand, there are often only small amount of data available that can be used to solve the inverse problem. To overcome these challenges, novel methods are needed. In Taylor mapping (TM), the solution of the ODE with respect to the initial values is developed into a polynomial series. The associated Taylor weights are computed using ODEs and are valid for each initial value problem of this ODE. The approach of TM can be used for the calculation of the solution of the inverse problem. The underlying ODE has not to be known explicitly. The Taylor weights are computed by solving a least square problem with respect to the discrete time values of the time series. This algorithm can be interpreted as a Polynomial Neural Network (PNN) where the neurons are polynomials, and the layers present a certain polynomial order. The Taylor Mapping and PNN approach is demonstrated and analyzed on academic examples. The accuracy and the forecast of the solution are in focus. Further, a catalytic reaction is presented as a realworld application.
Bio:
Uwe Iben is an engineer and applied mathematician at Central Research and Advance Engineering of Robert Bosch GmbH. He worked for more than 15 years as a chief expert on the field of cavitation and multi-phase flow and developed models and numerical schemes for simulation of cavitation and cavitation induced erosion. He is currently working as a chief expert on methods for solving differential equations using AI methods. Since 2017, he has been an honor professor at the University of Stuttgart.
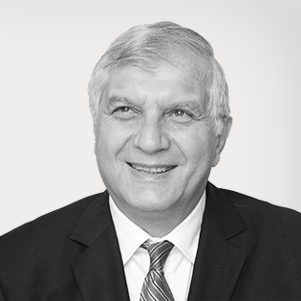
Engineering, big data, and the future
Abstract:
Advancements in technology and data access are followed by significant changes in the scope of engineering work. In Industry 4.0 sensor technologies enable improved monitoring, diagnostic, prognostic and prescriptive analytic capabilities providing systems and processes with a digital asset that parallels the physical assets. This evolution triggers a shift from engineering of design to engineering of performance. This change involves an integration of mathematical models, driven by physics, with statistical and analytic data driven models. In this context, differential and partial differential equations are combined with empirical models to provide enhanced precision and generalizability. Topics of current interest include hybrid models, soft sensors, digital twins and model order reduction methods. The talk will review this background and sketch future pathways emphasizing engineering of performance, in contrast to engineering of design. This conceptual shift repositions the role of model-based system engineering (MBSE) and detailed requirement analysis as systems are increasingly considered “porous” with upfront incomplete requirements. Particular attention will be given to systems of systems that are extremely large, extremely uncertain and extremely complex. The talk makes the case that the goal of engineering for performance is to achieve optimal decision-making with high accuracy in almost real-time. A case study from the Israeli railway system will be presented.
Bio:
Professor Ron Kenett is Senior Research Fellow at the Samuel Neaman Institute, Technion, Haifa, Israelת Chairman of the KPA Group, Israel, Chairman of the Data Science Society at AEAI and Research Professor at the University of Turin, Italy. He is an applied statistician combining expertise in academic, consulting and business domains. Ron is member of the Public Advisory Council for Statistics Israel, member of the of Digital Twin Center of Excellence (DiTCE) at Ben Gurion University of the Negev, member of the INFORMS QSR advisory board and member of the advisory board of DSRC, the University of Haifa Data Science Research Center. He is Past President of the Israel Statistical Association (ISA) and of the European Network for Business and Industrial Statistics (ENBIS). He authored and co-authored over 250 papers and 18 books on data science, industrial statistics, biostatistics, healthcare, customer surveys, multivariate quality control, risk management, system and software testing, and information quality. The KPA Group, he founded in 1994, is a leading Israeli firm focused on generating insights through analytics. He was awarded the 2013 Greenfield Medal by the Royal Statistical Society, in 2018, the George Box Medal by the European Network for Business and Industrial Statistics and, in 2022, the life achievement award by the Israeli Society for Quality. He earned a BSc in Mathematics (with first class honors) from Imperial College, London University and a PhD in Mathematics from the Weizmann Institute of Science, Rehovot, Israel.
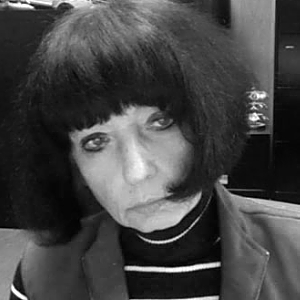
Can we control a flutter in flow-structure interactions?
Abstract:
Flow-structure interactions are ubiquitous in nature. Problems such as attenuation of turbulence or flutter in an oscillating structure [Tacoma bridge],flutter in tall buildings, fluid flows in flexible pipes, in nuclear engineering flows about fuel elements and heat exchanger vanes - are prime examples of relevant applications. Mathematically, the models are represented by a 3D compressible, irrotational Euler Equation coupled to a nonlinear dynamic elasticity on a 2D manifold. Strong boundary-type coupling at the interface between the two media is at the center of the analysis. This provides for a rich mathematical structure, opening the door to several unresolved problems in the area of nonlinear PDE’s, dynamical systems, related harmonic analysis and differential geometry. This talk aims at providing a brief overview of recent developments in the area along with a presentation of some recent advances addressing the issues of control and long time behavior of such models.
Bio:
Irena Lasiecka is the Distinguished University Professor at the University of Memphis and a former Commonwealth Professor at the University of Virginia. She received her PhD (1975) in Applied Mathematics from the University of Warsaw. She then spent 3 years at UCLA in a Post-Doc position, followed by Professorships at the University of Florida and the University of Virginia until 2013. She has also held numerous visiting appointments including Universite de Liege, the IMA at the University of Minnesota, the University of Bologna, UCLA, the Scuola Normale Superiore di Pisa, the University of Warsaw. Her research interests are in the general area of control theory for infinite dimensional systems - predominantly Partial Differential Equations (PDEs) with applications to interactive structures such as they arise in flutter control in aero-elasticity, turbulence suppression in fluids, noise attenuation in acoustic chambers, etc In this area she has published 5 research monographs and over 300 research papers. Her work was cited over 9,000 according to MathScnet and over 18,800 according to Google Scholar with H index =68. Her work has been funded by NSF, AFOSR, ARO and also NASA. She has been in the ISI’s List of Highly Cited researchers since its inception. She was an IEEE Distinguished Lecturer, and a frequent Plenary Speaker at SIAM, AMS, IFIP, AIMS Conferences. She served as an associate editor of many journals including: IEEE Transactions on Automatic Control, Automatica, Systems and Control Letters, Siam Journal on Control, Journal of Optimization Theory and Applications, Applied Mathematics and Optimization, Journal of Mathematical Analysis and Applications, Nonlinear Analysis, Discrete and Continuous Dynamical Systems.She is Editor-In-Chief [jointly with Roger Temam and Huyen Pham] of Applied Mathematics and Optimization [Springer] as well as of Evolution Equations and Control Theory [AIMS], jointly with Alain Haraux. She has been involved in leadership positions with IFIP [International Organization of Information Processes], including chair of the TC7-Committee on Modeling and Optimization. She has advised over 30 PhD students, several of whom have then pursued successful academic careers of their own and are now leaders in the field. She is a 2004 IEEE Fellow , 2019 SIAM Fellow and 2015 AMS Fellow. In 2011 she has was awarded the 2011 SIAM W.I. Reid Prize for contributions to control theory and differential equations. In 2019 she was awarded by AACC [American Automatic Control Council] the Richard Bellman Control Heritage Award for her contribution to boundary control of distributed parameter systems, 2020 William Sparks Eminent Faculty Award from Board of Trustees TN, and 2021 Hugo Steinhaus Award from Polish Mathematical Society. She was appointed Eisenbud Research Professor at MSRI, University of California, Berkelay for the semester on Mathematical Theory of Fluid Dynamics, 2021.
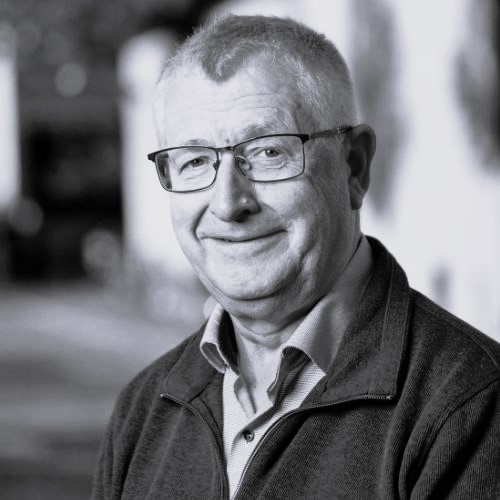
Forecasting for the Weather-Driven Energy System
Abstract:
The transition to a future weather-driven and low carbon society implies that the energy system will undergo a fundamental change from a system where the production follows demand to a system where the demand follows the renewable power production. This also implies that access to state-of-the-art methods for forecasting will become important. In this talk recent developments in methods for renewable energy forecasting will be described. We will focus on methods for multivariate probabilistic forecasting of load, prices and renewable power generation. It will be argued that tools for integrated forecasting across domains (wind, solar, load, prices, ...) will become essential, and replace more silo-oriented tools for individual areas like wind power. It will be demonstrated that full multivariate probabilistic forecasts are important to obtain reliability and profitability in the operation of the future low-carbon energy system. Real-life decision problems often involve forecasts for multiple levels of a hierarchy. For instance it becomes essential that reliable and forecasts on all grid levels are coherent such that e.g., forecasts provided for wind farm owners, the DSO (distribution system operator) and the TSO (transmission system operator) are aligned. Reconciliation ensures unified forecasts that support aligned decisions across all levels of a hierarchy, whether it is cross–sectional, temporal, or spatial. This talk describes some of the recent developments in forecasting using both spatial and temporal hierarchies.
Bio:
He got a PhD in Statistics at the Technical University of Denmark in 1986. He was appointed Ass. Prof. in Statistics in 1986, Assoc. Prof. in 1989, and Professor in Mathematical Statistics with a special focus on Stochastic Dynamical Systems in 1999. In 2017 he was appointed Professor II at NTNU in Trondheim. His main research interest is related to analysis and data-driven modelling of dynamical systems. This includes signal processing, time series analysis, model building, estimation, grey-box modelling, digital twins, prediction, optimization and control. The applications are mostly related to the digital transformation of the energy and water systems. He has got several awards. Lately, in June 2016, he was appointed Knight of the Order of Dannebrog by Her Majesty the Queen of Denmark, and he was appointed Doctor HC at Lund University in June 2017. He has authored or co-authored approximately 650 papers and 12 books. The most recent books are Time Series Analysis (2008); General and Generalized Linear Models (2011); Integrating Renewables in Electricity Markets (2013), and Statistics for Finance (2015).
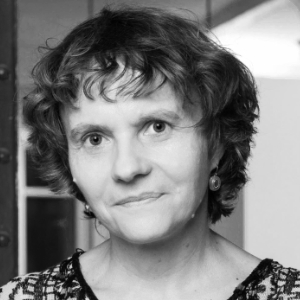
Machine Learning Design for Industrial Small Data regimes
Abstract:
In recent years, considerable progress has been made in the implementation of decision support procedures based on machine learning methods through the exploitation of very large databases and the use of learning algorithms. In the industrial environment, the databases available in research and development or in production are rarely so voluminous and the question arises as to whether in this context it is reasonable to want to develop powerful tools based on artificial learning techniques. This talk presents research work around transfer learning and hybrid models that use knowledge from related application domains or physics to implement efficient models with an economy of data. Several achievements in industrial collaborations will be presented that successfully use these learning models to design machine learning for industrial small data regimes and to develop powerful decision support tools even in cases where the initial data volume is limited.
Bio:
Mathilde Mougeot is Professeur of Data Science at Ecole Nationale Supérieure d'Informatique pour l'Industrie et l'Entreprise (ENSIIE) and adjunct Professor at ENS Paris Saclay where she holds the Industrial Research Chair "Industrial Data Analytics & Machine Learning". Since the beginning of her career, Mathilde Mougeot has been interested in machine learning for artificial intelligence applications. Her research activity is motivated by questions related to concrete applications stemming from collaborative projects with the socio-economic world. Her research focuses mainly on scientific issues related to predictive models in various contexts, such as those of high dimensionality, model aggregation, domain adaptation, data frugality by model transfer or by hybrid models. From 1999 to 2005, she has been contributed to the creation and the development of the start-up Miriad Technologies, specialized in the development of mathematical solutions for the industry based on machine learning, statistics and signal processing techniques. From 2016 to 2019, she was scientific officer for technology transfer in the Mathematics Division of Centre National de la Recherche Scientifique (CNRS) France. She offers a strong experience in leading projects at the interface of academics and industry.
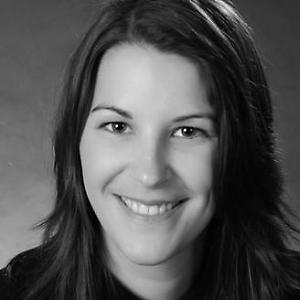
PDE-constrained Optimization under Uncertainty
Abstract:
Approaches to decision making and learning mainly rely on optimization techniques to achieve “best” values for parameters and decision variables. In most practical settings, however, the optimization takes place in the presence of uncertainty about model correctness, data relevance, and numerous other factors that influence the resulting solutions. For complex processes modeled by nonlinear ordinary and partial differential equations, the incorporation of these uncertainties typically results in high or even infinite dimensional problems in terms of the uncertain parameters as well as the optimization variables, which in many cases are not solvable with current state of the art methods. One promising potential remedy to this issue lies in the approximation of the forward problems using novel techniques arising in uncertainty quantification and machine learning. We propose in this talk a general framework for machine learning based optimization under uncertainty and inverse problems. Our approach replaces the complex forward model by a surrogate, e.g. a neural network, which is learned simultaneously in a one-shot sense when estimating the unknown parameters from data or solving the optimal control problem. By establishing a link to the Bayesian approach, an algorithmic framework is developed which ensures the feasibility of the parameter estimate / control w.r. to the forward model.
Bio:
Claudia is a Professor in Numerical analysis of stochastic and deterministic partial differential equations at Free University Berlin. Her research interests focus on the development of efficient methods for large-scale optimization problems in the presence of uncertainty in measurements, models, and parameters. Claudia received her PhD degree from the Department of Mathematics, University of Trier (Germany) in 2011. After two and half years of postdoctoral activity at ETH Zurich (Switzerland) and two years at the University of Warwick (UK), she was visiting professor at the Humboldt University Berlin (Germany) in 2015 - 2016 and then moved to Mannheim in 2017. In March 2022, she joined Free University Berlin. She has been working on a unified framework for the proper treatment of uncertainties in inverse and optimization problems for complex applications. Recent work includes the design of preconditioners for sampling techniques in the small noise or large data limit, data-informed approximations of the underlying model, and the analysis of particle-based methods for inverse problems.
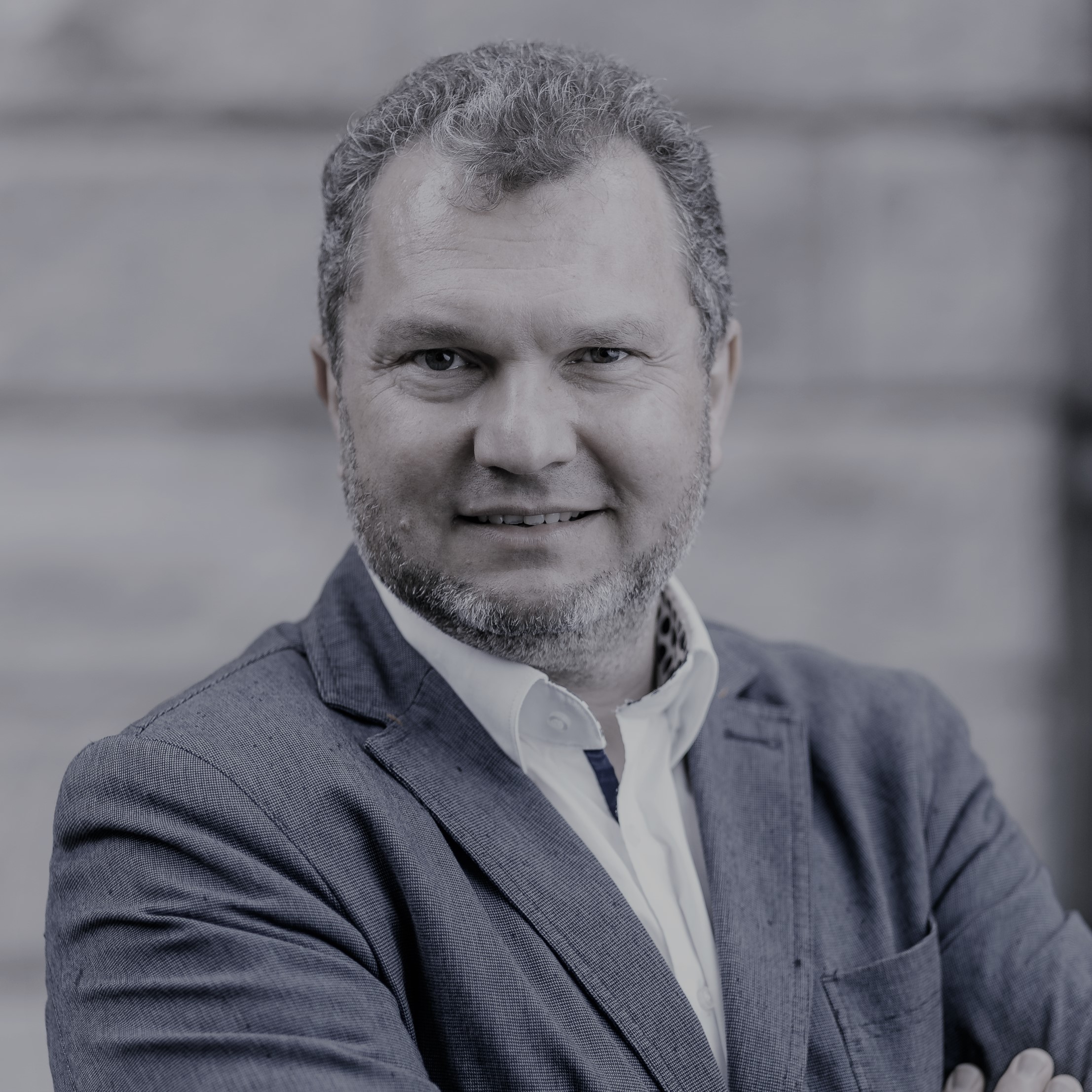
Recent Advances in Electricity Price Forecasting: A 2023 Perspective
Abstract:
Electricity price forecasting (EPF) is a branch of energy forecasting on the interface between econometrics/statistics, computer science and engineering, which focuses on predicting the spot and forward prices in wholesale electricity markets. Over the last 25 years, a variety of methods and ideas have been tried for EPF, with varying degrees of success. In this talk I will review recent developments in this fascinating area, including (but not limited to) probabilistic forecasting, combining forecasts and deep learning. .
Bio:
Rafal Weron is Professor of Management Science and Head of the Department of Operations Research and Business Intelligence at the Wroclaw University of Science and Technology. He is one of the leading world experts on energy forecasting and is periodically engaged as a consultant to financial, energy and software engineering companies. Details on current projects and publications are available on Rafal's website (https://p.wz.pwr.edu.pl/~weron.rafal/).